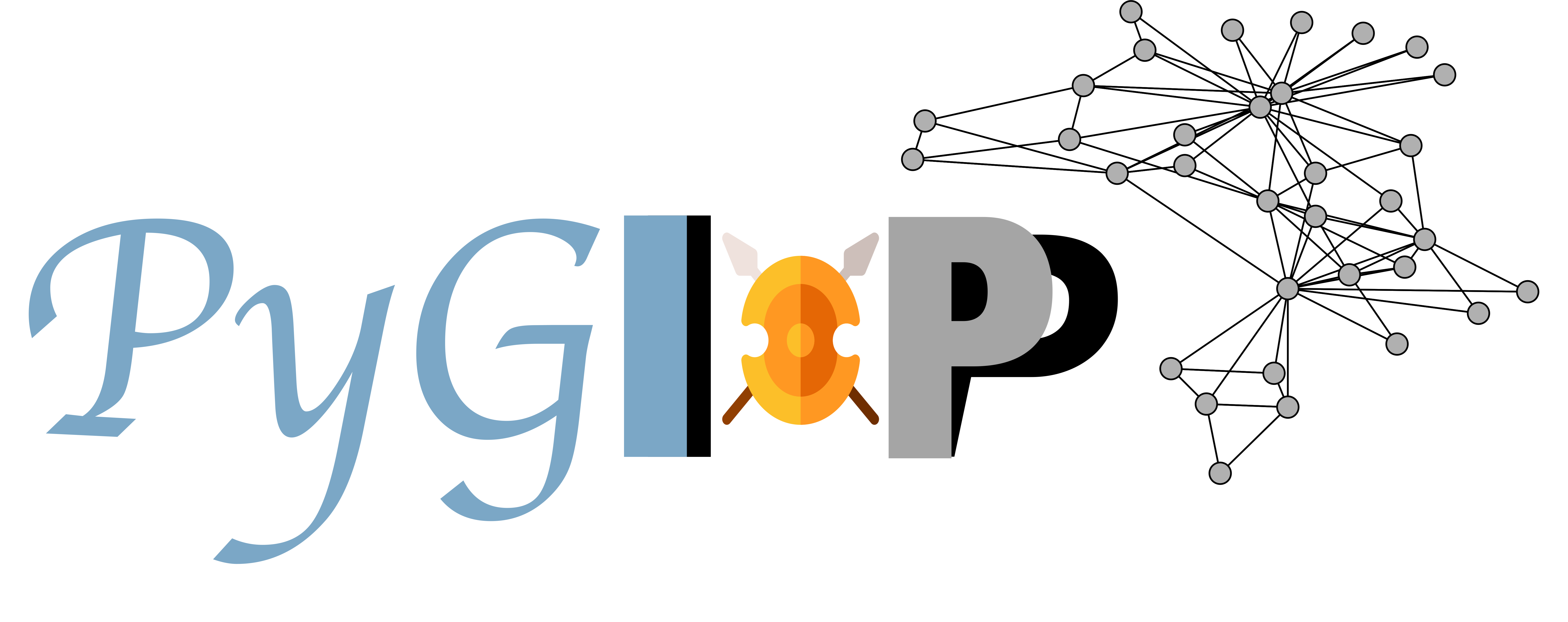
PyGIP is a comprehensive Python library focused on model extraction attacks and defenses in Graph Neural Networks (GNNs). Built on PyTorch, PyTorch Geometric, and DGL, the library offers a robust framework for understanding, implementing, and defending against attacks targeting GNN models.
PyGIP is featured for:
Extensive Attack Implementations: Multiple strategies for GNN model extraction attacks, including fidelity and accuracy evaluation.
Defensive Techniques: Tools for creating robust defense mechanisms, such as watermarking graphs and inserting synthetic nodes.
Unified API: Intuitive APIs for both attacks and defenses.
Integration with PyTorch/DGL: Seamlessly integrates with PyTorch Geometric and DGL for scalable graph processing.
Customizable: Supports user-defined attack and defense configurations.
Quick Start Example:
Outlier Detection Example with 5 Lines of Code:
from gnn_mae import ModelExtractionAttack2
dataset = ... # Load your graph dataset as a DGL object
attack = ModelExtractionAttack2(dataset, attack_node_fraction=0.25)
attack.attack()
# Evaluate fidelity and accuracy
print(f"Fidelity: {attack.fidelity}, Accuracy: {attack.accuracy}")
Attack Modules¶
Class Name |
Attack Type |
Reference |
---|---|---|
Model Extraction Attack; Black-Box |
DeFazio, D., & Ramesh, A. (2019). Adversarial model extraction on graph neural networks. arXiv preprint arXiv:1912.07721. |
|
Model Extraction Attack; Grey-Box |
Wu, B., Yang, X., Pan, S., & Yuan, X. (2022). Model extraction attacks on graph neural networks: Taxonomy and realisation. In Proceedings of the 2022 ACM on Asia conference on computer and communications security, 337-350. |
|
Model Extraction Attack; Black-Box |
Wu, B., Yang, X., Pan, S., & Yuan, X. (2022). Model extraction attacks on graph neural networks: Taxonomy and realisation. In Proceedings of the 2022 ACM on Asia conference on computer and communications security, 337-350. |
|
Model Extraction Attack; Black-Box |
Wu, B., Yang, X., Pan, S., & Yuan, X. (2022). Model extraction attacks on graph neural networks: Taxonomy and realisation. In Proceedings of the 2022 ACM on Asia conference on computer and communications security, 337-350. |
|
Model Extraction Attack; Black-Box |
Wu, B., Yang, X., Pan, S., & Yuan, X. (2022). Model extraction attacks on graph neural networks: Taxonomy and realisation. In Proceedings of the 2022 ACM on Asia conference on computer and communications security, 337-350. |
|
Model Extraction Attack; Grey-Box |
Wu, B., Yang, X., Pan, S., & Yuan, X. (2022). Model extraction attacks on graph neural networks: Taxonomy and realisation. In Proceedings of the 2022 ACM on Asia conference on computer and communications security, 337-350. |
|
Model Extraction Attack; Grey-Box |
Wu, B., Yang, X., Pan, S., & Yuan, X. (2022). Model extraction attacks on graph neural networks: Taxonomy and realisation. In Proceedings of the 2022 ACM on Asia conference on computer and communications security, 337-350. |
|
Model Extraction Attack; Black-Box |
Shen, Y., He, X., Han, Y., & Zhang, Y. (2022). Model stealing attacks against inductive graph neural networks. In 2022 IEEE Symposium on Security and Privacy (SP), 1175-1192. |
|
*Grey-box attacks assume the attacker has at least partial knowledge across all dimensions (i.e., node attributes, graph structure, and shadow graph). | ||
**Black-box attacks refer to scenarios where the attacker has no knowledge regarding at least one dimension (i.e., node attributes, graph structure, or shadow graph). |
Defense Modules¶
Class Name |
Defense Type |
Reference |
---|---|---|
Watermarking-based defense technology. |
Zhao, X., Wu, H., & Zhang, X. (2021). Watermarking graph neural networks by random graphs. In 2021 9th International Symposium on Digital Forensics and Security (ISDFS), 1-6. |
Additional Details¶
For further information, refer to individual classes like GraphNeuralNetworkMetric, WatermarkGraph, or the specific model extraction attacks in the source code.